17.3. 可観測性
17.3.1. OpenShift Container Platform における可観測性
OpenShift Container Platform では、プラットフォームとプラットフォーム上で実行されているワークロードの両方から、パフォーマンスメトリクスやログなどの大量のデータが生成されます。管理者は、さまざまなツールを使用して、利用可能なすべてのデータを収集および分析できます。以下は、可観測性スタックを設定するシステムエンジニア、アーキテクト、および管理者向けのベストプラクティスの概要です。
特に明記のない限り、このドキュメントの内容はエッジデプロイメントとコアデプロイメントの両方を表しています。
17.3.1.1. モニタリングスタックについて
モニタリングスタックは次のコンポーネントを使用します。
- Prometheus は、OpenShift Container Platform コンポーネントおよびワークロードからメトリクスを収集して分析します (そのように設定されている場合)。
- Alertmanager は、アラートのルーティング、グループ化、およびサイレンスを処理する Prometheus のコンポーネントです。
- Thanos はメトリクスの長期保存を処理します。
図17.2 OpenShift Container Platform モニタリングアーキテクチャー
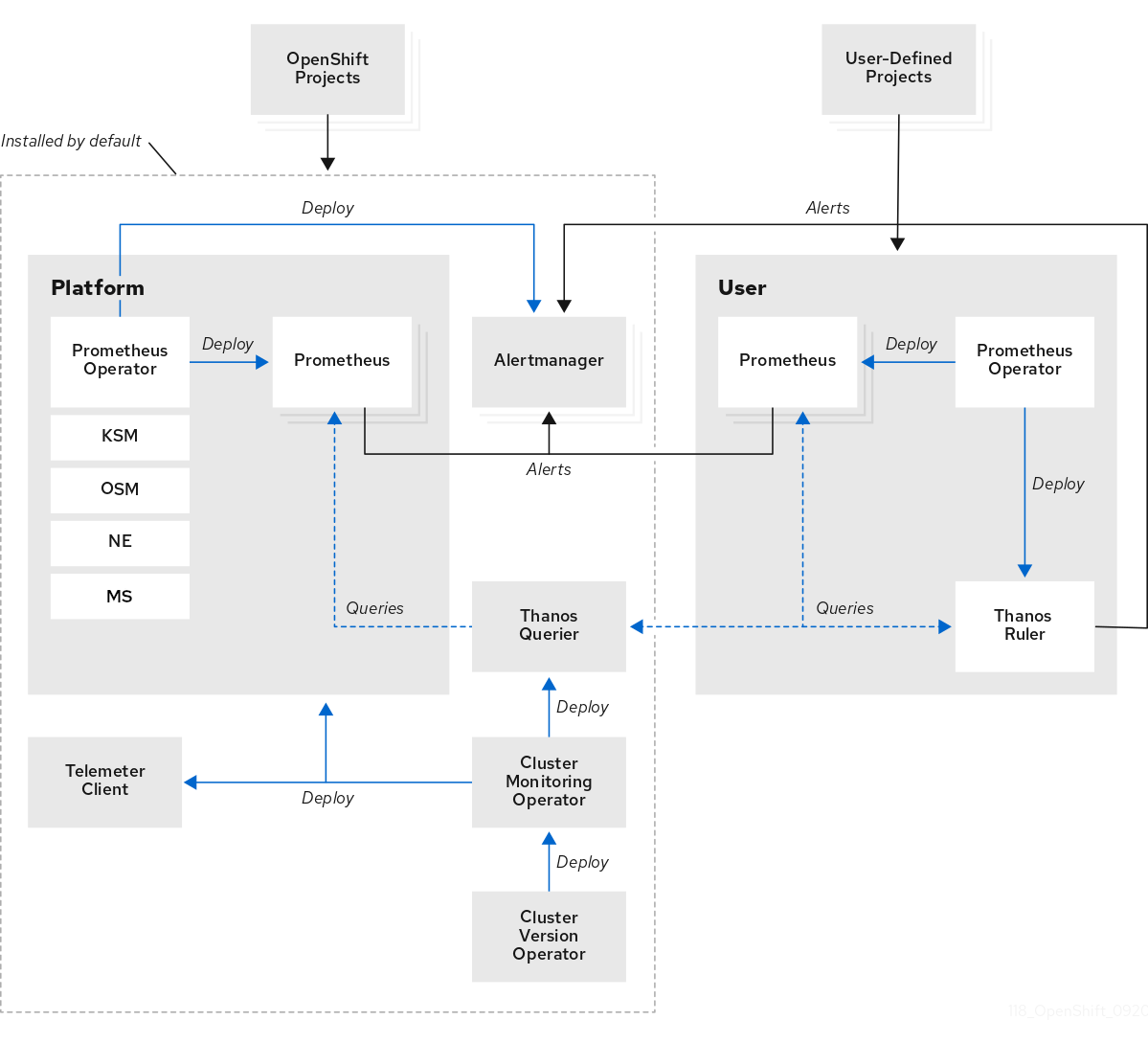
シングルノード OpenShift クラスターの場合、クラスターは分析と保持のためにすべてのメトリクスをハブクラスターに送信するため、Alertmanager と Thanos を無効にする必要があります。
17.3.1.2. 主要なパフォーマンスメトリクス
システムによっては、利用可能な測定値が数百種類ある場合があります。
注目すべき重要なメトリクスは次のとおりです。
-
etcd
応答時間 - API 応答時間
- Pod の再起動とスケジュール
- リソースの使用状況
- OVN の健全性
- クラスター Operator の全体的な健全性
原則として、あるメトリクスが重要であると判断した場合は、それに対するアラートを設定することを推奨します。
次のコマンドを実行すると、利用可能なメトリクスを確認できます。
$ oc -n openshift-monitoring exec -c prometheus prometheus-k8s-0 -- curl -qsk http://localhost:9090/api/v1/metadata | jq '.data
17.3.1.2.1. PromQL のクエリー例
次の表は、OpenShift Container Platform コンソールを使用してメトリクスクエリーブラウザーで調べることができるクエリーの一部を示しています。
コンソールの URL は https://<OpenShift Console FQDN>/monitoring/query-browser です。次のコマンドを実行すると、Openshift コンソールの FQDN を取得できます。
$ oc get routes -n openshift-console console -o jsonpath='{.status.ingress[0].host}'
メトリクス | Query |
---|---|
|
|
|
|
|
|
|
|
|
|
|
|
合計 |
|
メトリクス | Query |
---|---|
|
|
|
|
リーダー選出 |
|
ネットワークレイテンシー |
|
メトリクス | Query |
---|---|
デグレード状態の Operator |
|
クラスターあたりのデグレード状態の Operator 総数 |
|
17.3.1.2.2. メトリクスの保存に関する推奨事項
デフォルトでは、Prometheus は保存されたメトリクスを永続ストレージにバックアップしません。Prometheus Pod を再起動すると、すべてのメトリクスデータが失われます。プラットフォームで使用可能なバックエンドストレージを使用するようにモニタリングスタックを設定する必要があります。Prometheus の高い IO 要求を満たすには、ローカルストレージを使用する必要があります。
通信事業者向けコアクラスターの場合、Prometheus の永続ストレージに Local Storage Operator を使用できます。
ブロック、ファイル、およびオブジェクトストレージ用の Ceph クラスターをデプロイする Red Hat OpenShift Data Foundation (ODF) も、通信事業者向けコアクラスターの候補として適しています。
RAN シングルノード OpenShift またはファーエッジクラスターのシステムリソース要件を低く抑えるには、モニタリングスタック用のバックエンドストレージをプロビジョニングしないでください。このようなクラスターは、すべてのメトリクスをハブクラスターに転送します。そこにサードパーティーのモニタリングプラットフォームをプロビジョニングできます。
17.3.1.3. エッジのモニタリング
シングルノード OpenShift をエッジに配置することで、プラットフォームコンポーネントのフットプリントが最小限に抑えられます。次の手順は、モニタリングフットプリントが小さいシングルノード OpenShift ノードを設定する方法の例です。
前提条件
- Red Hat Advanced Cluster Management (RHACM) を使用する環境で、可観測性サービスが有効になっている。
- ハブクラスターで Red Hat OpenShift Data Foundation (ODF) が実行されている。
手順
次の例のように、
ConfigMap
CR を作成し、monitoringConfigMap.yaml
として保存します。apiVersion: v1 kind: ConfigMap metadata: name: cluster-monitoring-config namespace: openshift-monitoring data: config.yaml: | alertmanagerMain: enabled: false telemeterClient: enabled: false prometheusK8s: retention: 24h
シングルノード OpenShift で、次のコマンドを実行して
ConfigMap
CR を適用します。$ oc apply -f monitoringConfigMap.yaml
次の例のように、
NameSpace
CR を作成し、monitoringNamespace.yaml
として保存します。apiVersion: v1 kind: Namespace metadata: name: open-cluster-management-observability
ハブクラスターで、次のコマンドを実行して、ハブクラスターに
Namespace
CR を適用します。$ oc apply -f monitoringNamespace.yaml
次の例のように、
ObjectBucketClaim
CR を作成し、monitoringObjectBucketClaim.yaml
として保存します。apiVersion: objectbucket.io/v1alpha1 kind: ObjectBucketClaim metadata: name: multi-cloud-observability namespace: open-cluster-management-observability spec: storageClassName: openshift-storage.noobaa.io generateBucketName: acm-multi
ハブクラスターで、次のコマンドを実行して
ObjectBucketClaim
CR を適用します。$ oc apply -f monitoringObjectBucketClaim.yaml
次の例のように、
Secret
CR を作成し、monitoringSecret.yaml
として保存します。apiVersion: v1 kind: Secret metadata: name: multiclusterhub-operator-pull-secret namespace: open-cluster-management-observability stringData: .dockerconfigjson: 'PULL_SECRET'
ハブクラスターで、次のコマンドを実行して
Secret
CR を適用します。$ oc apply -f monitoringSecret.yaml
次のコマンドを実行して、ハブクラスターから NooBaa サービスのキーとバックエンドバケット名を取得します。
$ NOOBAA_ACCESS_KEY=$(oc get secret noobaa-admin -n openshift-storage -o json | jq -r '.data.AWS_ACCESS_KEY_ID|@base64d')
$ NOOBAA_SECRET_KEY=$(oc get secret noobaa-admin -n openshift-storage -o json | jq -r '.data.AWS_SECRET_ACCESS_KEY|@base64d')
$ OBJECT_BUCKET=$(oc get objectbucketclaim -n open-cluster-management-observability multi-cloud-observability -o json | jq -r .spec.bucketName)
次の例のように、バケットストレージ用の
Secret
CR を作成し、monitoringBucketSecret.yaml
として保存します。apiVersion: v1 kind: Secret metadata: name: thanos-object-storage namespace: open-cluster-management-observability type: Opaque stringData: thanos.yaml: | type: s3 config: bucket: ${OBJECT_BUCKET} endpoint: s3.openshift-storage.svc insecure: true access_key: ${NOOBAA_ACCESS_KEY} secret_key: ${NOOBAA_SECRET_KEY}
ハブクラスターで、次のコマンドを実行して
Secret
CR を適用します。$ oc apply -f monitoringBucketSecret.yaml
次の例のように、
MultiClusterObservability
CR を作成し、monitoringMultiClusterObservability.yaml
として保存します。apiVersion: observability.open-cluster-management.io/v1beta2 kind: MultiClusterObservability metadata: name: observability spec: advanced: retentionConfig: blockDuration: 2h deleteDelay: 48h retentionInLocal: 24h retentionResolutionRaw: 3d enableDownsampling: false observabilityAddonSpec: enableMetrics: true interval: 300 storageConfig: alertmanagerStorageSize: 10Gi compactStorageSize: 100Gi metricObjectStorage: key: thanos.yaml name: thanos-object-storage receiveStorageSize: 25Gi ruleStorageSize: 10Gi storeStorageSize: 25Gi
ハブクラスターで、次のコマンドを実行して
MultiClusterObservability
CR を適用します。$ oc apply -f monitoringMultiClusterObservability.yaml
検証
次のコマンドを実行して、namespace 内のルートと Pod を確認し、サービスがハブクラスターにデプロイされていることを確認します。
$ oc get routes,pods -n open-cluster-management-observability
出力例
NAME HOST/PORT PATH SERVICES PORT TERMINATION WILDCARD route.route.openshift.io/alertmanager alertmanager-open-cluster-management-observability.cloud.example.com /api/v2 alertmanager oauth-proxy reencrypt/Redirect None route.route.openshift.io/grafana grafana-open-cluster-management-observability.cloud.example.com grafana oauth-proxy reencrypt/Redirect None 1 route.route.openshift.io/observatorium-api observatorium-api-open-cluster-management-observability.cloud.example.com observability-observatorium-api public passthrough/None None route.route.openshift.io/rbac-query-proxy rbac-query-proxy-open-cluster-management-observability.cloud.example.com rbac-query-proxy https reencrypt/Redirect None NAME READY STATUS RESTARTS AGE pod/observability-alertmanager-0 3/3 Running 0 1d pod/observability-alertmanager-1 3/3 Running 0 1d pod/observability-alertmanager-2 3/3 Running 0 1d pod/observability-grafana-685b47bb47-dq4cw 3/3 Running 0 1d <...snip…> pod/observability-thanos-store-shard-0-0 1/1 Running 0 1d pod/observability-thanos-store-shard-1-0 1/1 Running 0 1d pod/observability-thanos-store-shard-2-0 1/1 Running 0 1d
- 1
- リスト表示される Grafana ルートからダッシュボードにアクセスできます。これを使用して、すべてのマネージドクラスターのメトリクスを表示できます。
Red Hat Advanced Cluster Management の可観測性の詳細は、可観測性 を参照してください。
17.3.1.4. アラート
OpenShift Container Platform には多数のアラートルールが含まれています。ルールはリリースごとに変更される可能性があります。
17.3.1.4.1. デフォルトのアラートの表示
クラスター内のすべてのアラートルールを確認するには、次の手順を使用します。
手順
クラスター内のすべてのアラートルールを確認するには、次のコマンドを実行します。
$ oc get cm -n openshift-monitoring prometheus-k8s-rulefiles-0 -o yaml
ルールには説明を含めることができ、追加情報や軽減策へのリンクを提供できます。たとえば、
etcdHighFsyncDurations
のルールは次のとおりです。- alert: etcdHighFsyncDurations annotations: description: 'etcd cluster "{{ $labels.job }}": 99th percentile fsync durations are {{ $value }}s on etcd instance {{ $labels.instance }}.' runbook_url: https://github.com/openshift/runbooks/blob/master/alerts/cluster-etcd-operator/etcdHighFsyncDurations.md summary: etcd cluster 99th percentile fsync durations are too high. expr: | histogram_quantile(0.99, rate(etcd_disk_wal_fsync_duration_seconds_bucket{job=~".*etcd.*"}[5m])) > 1 for: 10m labels: severity: critical
17.3.1.4.2. アラート通知
アラートは OpenShift Container Platform コンソールで表示できますが、アラートを転送する外部レシーバーを設定することが管理者には推奨されます。OpenShift Container Platform は、次のレシーバータイプをサポートしています。
- PagerDuty: サードパーティーのインシデント対応プラットフォーム
- Webhook: POST リクエストを介してアラートを受信し、必要なアクションを実行できる任意の API エンドポイント
- Email: 指定されたアドレスにメールを送信する
- Slack: Slack チャネルまたは個々のユーザーに通知を送信する
関連情報
17.3.1.5. ワークロードのモニタリング
デフォルトでは、OpenShift Container Platform はアプリケーションワークロードのメトリクスを収集しません。ワークロードメトリクスを収集するようにクラスターを設定できます。
前提条件
- クラスターのワークロードメトリクスを収集するためのエンドポイントを定義した。
手順
次の例のように、
ConfigMap
CR を作成し、monitoringConfigMap.yaml
として保存します。apiVersion: v1 kind: ConfigMap metadata: name: cluster-monitoring-config namespace: openshift-monitoring data: config.yaml: | enableUserWorkload: true 1
- 1
true
に設定してワークロードのモニタリングを有効にします。
次のコマンドを実行して
ConfigMap
CR を適用します。$ oc apply -f monitoringConfigMap.yaml
次の例のように、
ServiceMonitor
CR を作成し、monitoringServiceMonitor.yaml
として保存します。apiVersion: monitoring.coreos.com/v1 kind: ServiceMonitor metadata: labels: app: ui name: myapp namespace: myns spec: endpoints: 1 - interval: 30s port: ui-http scheme: http path: /healthz 2 selector: matchLabels: app: ui
次のコマンドを実行して、
ServiceMonitor
CR を適用します。$ oc apply -f monitoringServiceMonitor.yaml
Prometheus はデフォルトでパス /metrics
をスクレイピングしますが、カスタムパスを定義することもできます。重要であると判断したメトリクスとともに、このエンドポイントをスクレイピング用に公開するかどうかは、アプリケーションのベンダー次第です。
17.3.1.5.1. ワークロードのアラートの作成
クラスター上のユーザーワークロードに対するアラートを有効にできます。
手順
次の例のように、
ConfigMap
CR を作成し、monitoringConfigMap.yaml
として保存します。apiVersion: v1 kind: ConfigMap metadata: name: cluster-monitoring-config namespace: openshift-monitoring data: config.yaml: | enableUserWorkload: true 1 # ...
- 1
true
に設定してワークロードのモニタリングを有効にします。
次のコマンドを実行して
ConfigMap
CR を適用します。$ oc apply -f monitoringConfigMap.yaml
次の例のように、アラートルール用の YAML ファイル (
monitoringAlertRule.yaml
) を作成します。apiVersion: monitoring.coreos.com/v1 kind: PrometheusRule metadata: name: myapp-alert namespace: myns spec: groups: - name: example rules: - alert: InternalErrorsAlert expr: flask_http_request_total{status="500"} > 0 # ...
次のコマンドを実行してアラートルールを適用します。
$ oc apply -f monitoringAlertRule.yaml